Mass adoption of generative AI hinges on improving processing efficiency and lowering total cost of ownership. Similar to the internet and the invention of its World Wide Web application, generative AI has seized the public’s imagination. ChatGPT, the most popular AI chatbot launched a mere eight months ago, caught the world by surprise, reported to be the fastest growing app in history, reaching 100 million users within the first two months of its existence.
Generative AI is gaining attention across all industry sectors with the promise of unleashing a wave of unparalleled productivity. The potential is massive, from assisting drug discovery and increasing the veracity of a doctor’s medical opinion to improving the accuracy of order delivery estimates and helping programmers write more efficient software code. It is expected to be deployed in about 70% of all work activities, supplementing revenues in excess of US$4 trillion.
Still, much more work needs to be done within the infrastructure. Unlike most applications, ChatGPT software is on track; the supporting hardware that runs the applications is not.
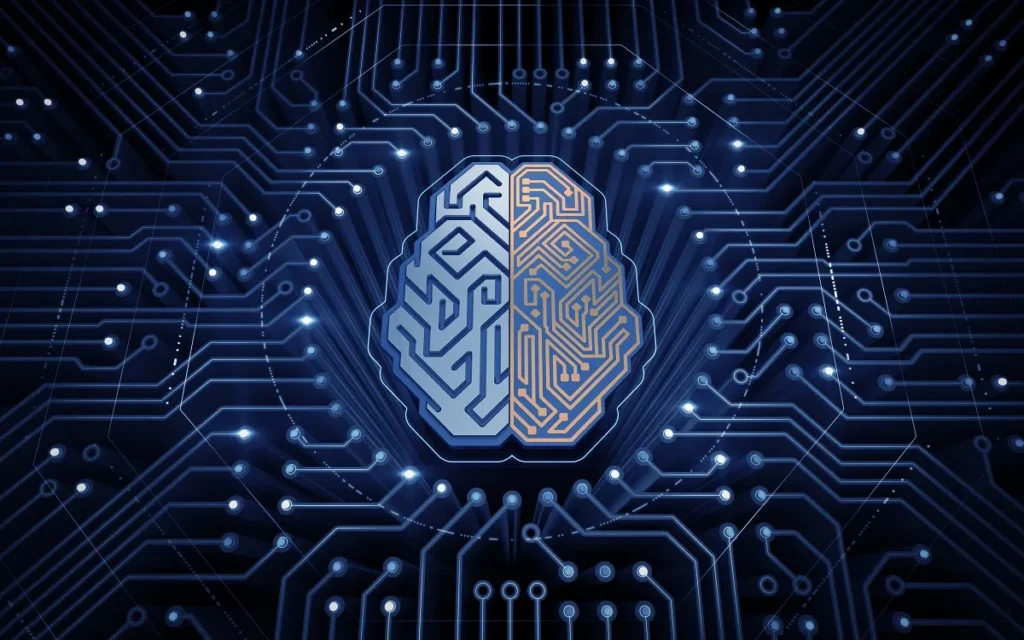
The hardware challenge with ChatGPT and other generative AI large-language models stems from the enormous number of parameters used by the algorithms to produce acceptable results. GPT-3.5, the previous generation of ChatGPT, required a staggering 175 billion parameters. Although not official yet, the number of parameters in the current GPT-4 version is estimated to increase tenfold to 1.7 trillion. AI hardware accelerators must scale to handle from 175 billion to almost 2 trillion parameters stored in memory to execute each user query.
Current computing architectures are not designed to handle that amount of data traffic between processor cores and memories, typically implemented outside computing cores. The setup leads to memory bottlenecks, commonly referred to as the “memory wall,” resulting in severe bandwidth limitations.
These factors rule out not only central processing units (CPUs), abysmally inadequate for the task, but also other AI computing architectures, such as graphics processing units (GPUs), as they cause processors to idle for most of the time, waiting to receive data. With GPT-4, leading GPUs idle for about 97% of the time. That equates to about 3% efficiency. At this low efficiency, a processor with a nominal computing power of 1 petaops—1015, or 1,000,000,000,000,000 operations per second—produces only about 30 teraops.
Today, these algorithms are executed on high-performance computing clusters, each consuming kilowatts of power. The ensuing problem: The actual power consumption to perform ChatGPT-4 user queries on a global scale goes off the charts, overloading power-generation plants and overstressing energy-distribution networks.
ChatGPT running cost
ChatGPT’s energy consumption is mind-boggling, but that’s not all. Its rapid rollout hampers the investment cost to acquire the hardware. Based on current purchasing options for leading processors, a back-of-the-envelope estimation shows that the acquisition cost of a GPT-4 processing system running 100,000 queries/second would be in the ballpark of hundreds of billions of U.S. dollars, while the energy costs of running it would be in the range of hundreds of millions of U.S. dollars per year.
Clearly, the cost is inconceivable and a roadblock to mass deployment of this innovative technology.
Meeting the requirements
This scenario offers a historical opportunity to the semiconductor industry to step up with supporting hardware. What is needed is a workable solution with three attributes to address the inadequate infrastructure architecture currently available to:
- Increase processing efficiencies from 2% to 4% to at least 50%
- A “theoretical” 1-petaops AI processor must deliver at least 500 teraops under real-life workloads.
- Lower the cost of hardware
- An “effective,” not “theoretical,” 1-petaops AI processor must cost no more than US$10,000.
- Lower power consumption
- An “effective,” not “theoretical,” 1-petaops AI processor must consume no more than 100 W.
Overall, to become economically sustainable as well as energy-efficient, a ChatGPT processing system must reach more than two orders of magnitude better cost-effectiveness. Only by lowering the total annual cost to run 100,000 queries/second on a GPT-4 system from hundreds of billions of U.S. dollars to less than US$10 billion will the promise of generative AI be delivered.